AIs and neuromorphic processors: Will we be able to handle the 'exponential gap'?
Is it now possible to partly reproduce the potential of the human brain in terms of computation, harnessing its speed and energy efficiency, due to the expanding knowledge of neural networks and neurobiology. But are we really capable of handling all this very rapid progress?
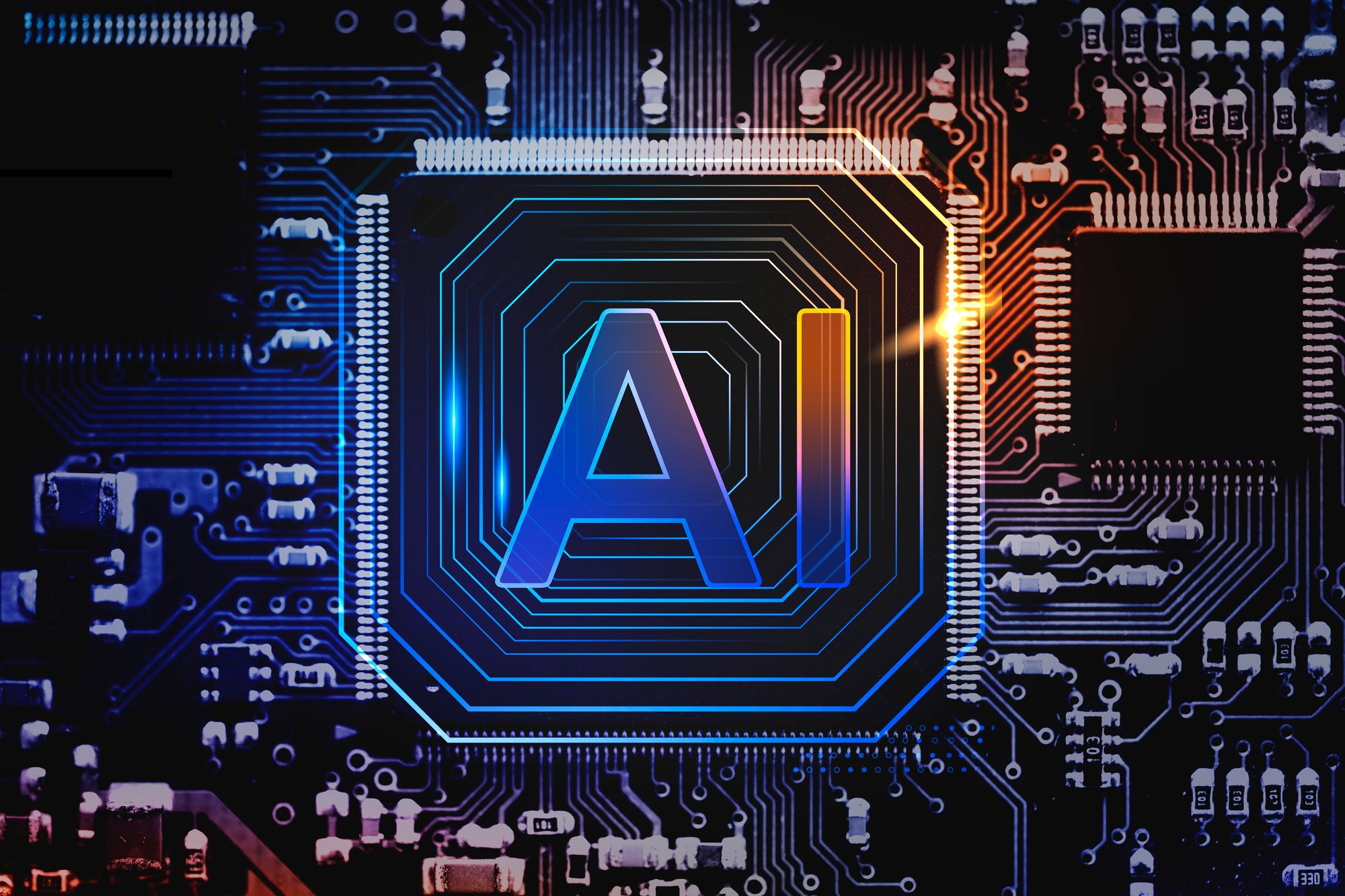
The human brain has a complex architecture and mode of computation and reasoning that can solve problems quickly and with controlled energy efficiency. Is it now possible to partly reproduce the potential of the human brain in terms of computation, harnessing its speed and energy efficiency, due to the expanding knowledge of neural networks and neurobiology. But are we really capable of handling all this very rapid progress?
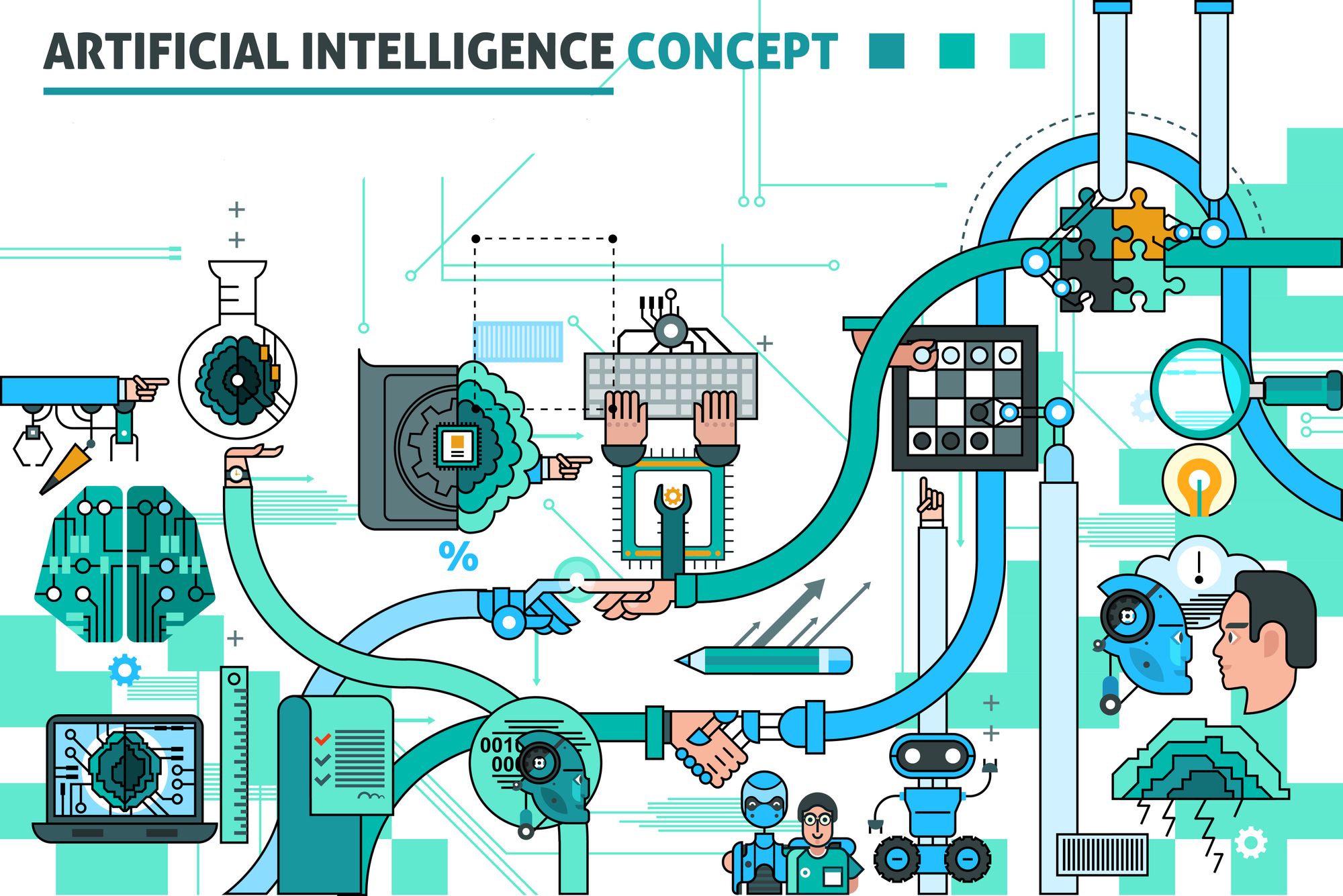
Human Neural Networks and AIs
Complex architecture, speed, and energy efficiency: these are the winning characteristics of our brains. The deepening understanding of how human neural networks and synapses work has opened up the vision of creating machines that can replicate the brain's computational power. Is it possible to talk about artificial intelligence in these terms? What has been unfolding before our eyes in the last few years (even months) is a vision of a prospect that is on the one hand frightening and on the other hand exciting because of the immense possibilities it opens up. The massive use of AI did not begin today; it was already present in seemingly simple operations, such as face and voice recognition. But today, the use of AI (especially those that generate content that does not require a specific degree of user specialization) has enabled a technological advance that was unthinkable until recently.
Convolutional Networks
Neuromorphic engineering, which has always been dedicated to studying and developing computational circuits that mimic the human brain down to its most intimate and specific aspects: Neuronal spiking, neural network architecture, and learning rules through synaptic plasticity, is also behind this concretization of the prospects for massive use of artificial intelligence. Deep learning is the backbone of many AI tools. It is widely implemented in social networks, self-driving cars, and virtual assistants. In particular, a specific category of multilayer networks, known as convolutional networks, is largely responsible for image recognition. [Convolutional neural network, CNN, LeCun, Y., Boser, B. E., Denker, J. S., Henderson, D., Howard, R. E., Hubbard, W. E., Jackel, L. D. (1990)]
In CNN, the input image is passed through a series of filters, each optimized to detect the presence of features such as lines, corners, and other more complex shapes. The success of CNN in image recognition can be attributed to the sharing of many artificial synapses in the feature search, which allows both learning and recognition to be performed with far fewer synapses than previous approaches. [LeCun, Y., Bengio, Y., Hinton, G. (2015). “Deep learning”, Nature, 521, 436-444.]
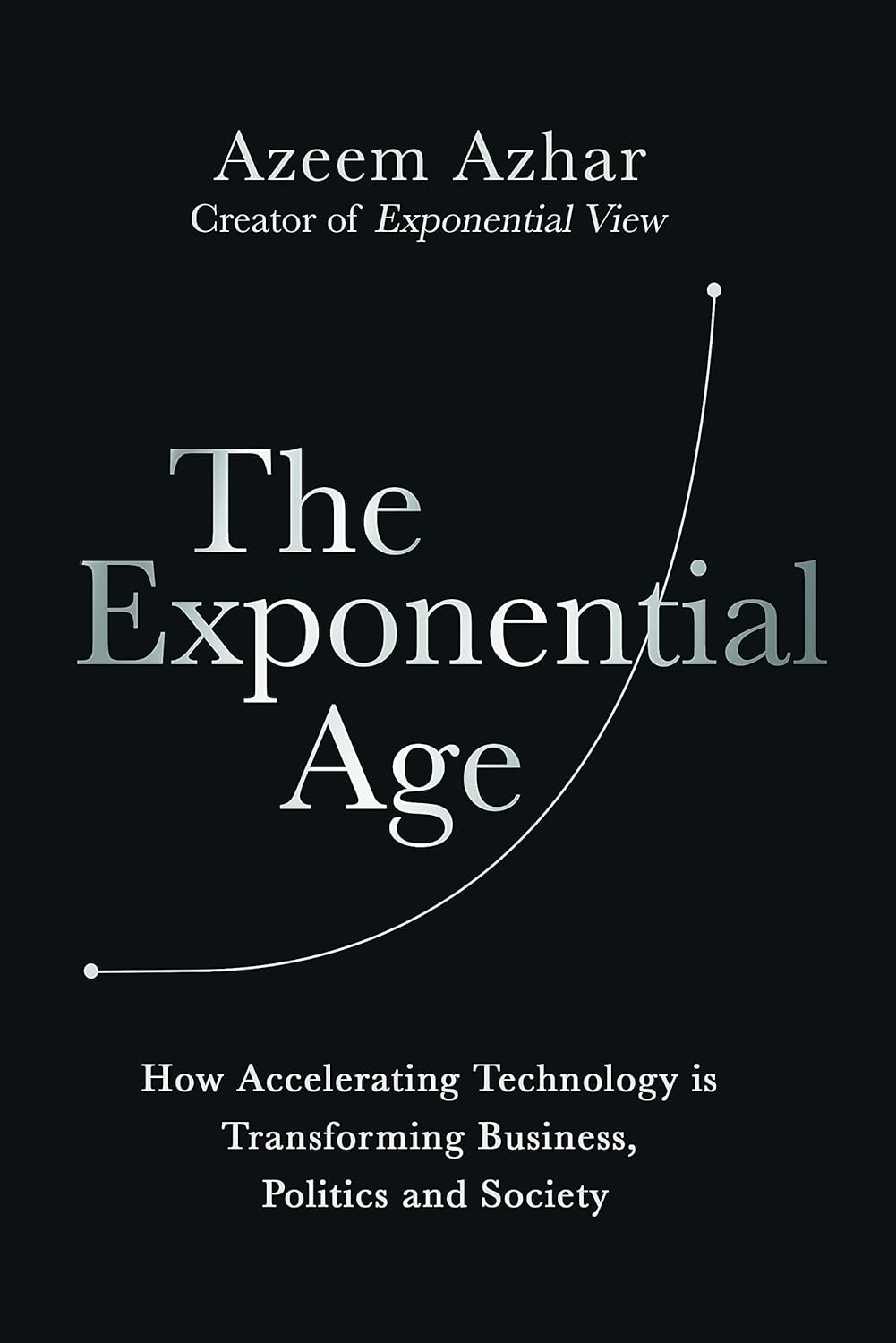
Management and Meanings
CNN is the basis for many of today's AI operations. It is an image classification method. CNN can recognize objects in a generic context, make connections and classifications, and this is fundamental to the "computer vision" technology of robotics. It is particularly suited to the context of automation and virtualization of symbols management and related meanings. An AI must be able to automatically interpret scenarios, to recognize the meaning related to the situation, to reproduce it by imitating its basic features (revising them if necessary), to structure what it interprets and to link it to all the elements that it has. Think of the video and the images generated today.
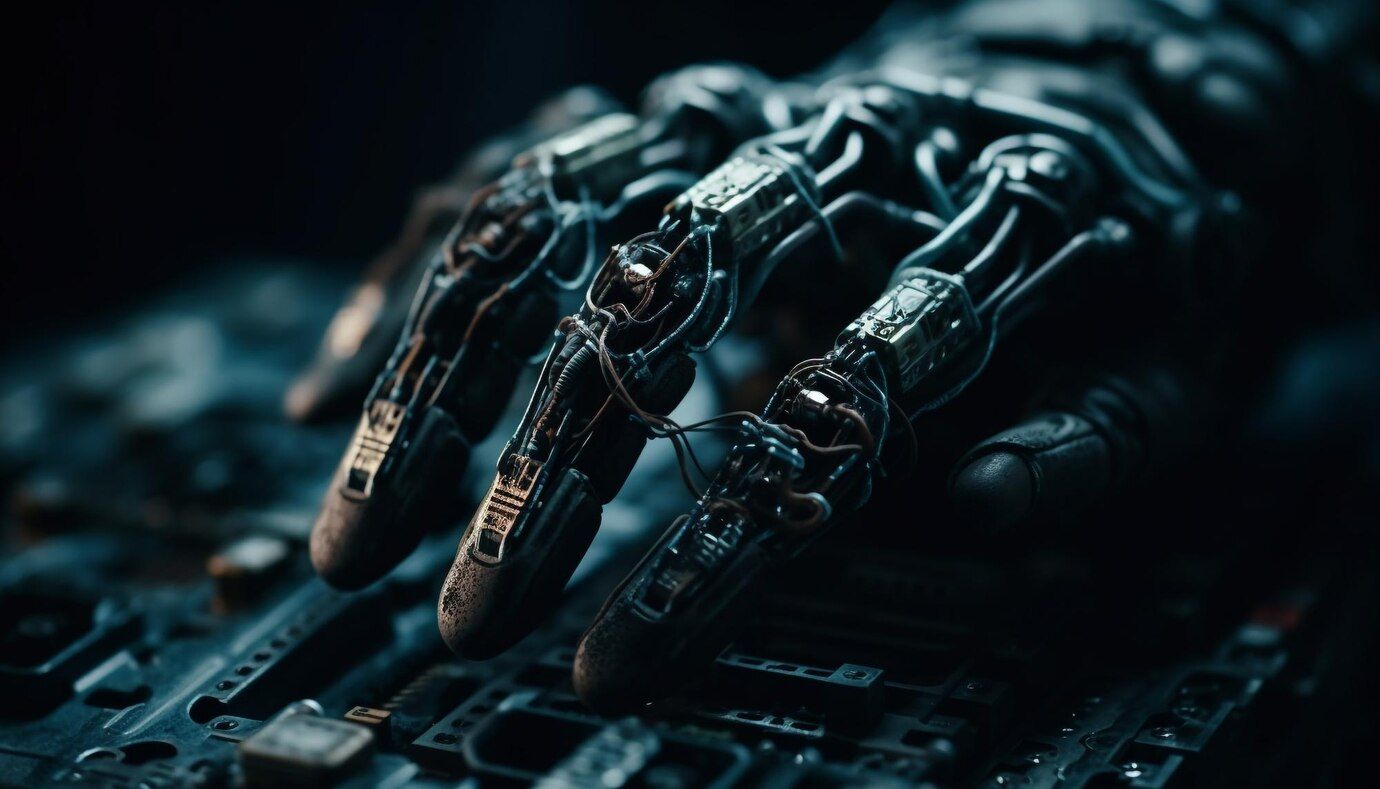
Managing I-Thought
There is no learning machine today that can do without a large data store, instructed by human operators to classify each piece of data with its label. But what if an "AI brain" began to function in every way like a human brain? A neuromorphic circuit would have to mimic the computational properties of the human brain, its sparse and hyper-connected neural network architecture, information spiking and asynchrony, and synaptic plasticity. In particular, synaptic plasticity could (and already does) affect learning mechanisms. Deep machine learning is still not like biological learning processes. However, synaptic plasticity applied to AI learning reproduce mechanisms typical of the human brain. They are true neuromorphic processors. They are capable of solving complex and unconventional problems, managing I-thought constraints, and using energy efficiently. The AIs we interact with today are already based on these principles and this technological approach. And for some, they are true personal assistants, able to lighten the workload and even interact in everyday life.
Hardware implementations of spiking neurons can be extremely useful for a large variety of applications, ranging from high-speed modeling of large-scale neural systems to real-time behaving systems, to bidirectional brain–machine interfaces. The specific circuit solutions used to implement silicon neurons depend on the application requirements. In this paper we describe the most common building blocks and techniques used to implement these circuits, and present an overview of a wide range of neuromorphic silicon neurons, which implement different computational models, ranging from biophysically realistic and conductance-based Hodgkin–Huxley models to bi-dimensional generalized adaptive integrate and fire models. We compare the different design methodologies used for each silicon neuron design described, and demonstrate their features with experimental results, measured from a wide range of fabricated VLSI chips [Here].
The Exponential Gap
But can these neuromorphic circuit systems outperform human models? In areas where strong and important decisions are required: finance, politics, medicine, can they replace humans?
In his book 'The Exponential Age', Azeem Azhar explains what he calls the "exponential gap." This is the gap between very rapid technological evolution and the ability of societies to adapt to that particular change. The question is, do we really have the capacity to adapt without being profoundly affected and changed as human beings by such transformative technology?
Insights and bibliography
- Azeem Azhar (2021). 'The Exponential Age: How Accelerating Technology is Transforming Business, Politics and Society'. Diversion Book.
- Daniele Ielmini (2018). 'Intelligenza artificiale: l'approccio neuromorfico'. Mondo Digitale.
- McCulloch, W. S., Pitts, W. A. (1943). 'A logical calculus of the ideas immanent in nervous activity'. Bulletin of Mathematical Biophysics, 5.
- LeCun, Y., Bengio, Y., Hinton, G. (2015). 'Deep learning'. Nature, 521.
- LeCun, Y., Boser, B. E., Denker, J. S., Henderson, D., Howard, R. E., Hubbard, W. E., Jackel, L. D. (1990). 'Handwritten digit recognition with a back-
propagation network', in Advances in Neural Information Processing Systems
(NIPS 1989), Denver (CO) (Vol. 2). Morgan Kaufmann.