AI: Let's call them artificial, but not intelligent
We should not think that everything an AI does is correct just because an AI does it. That is not true. It is not a logical system, but statistical.
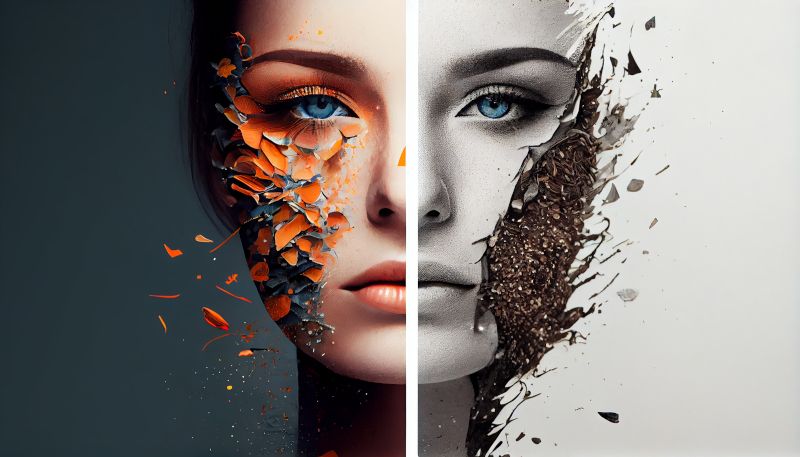
AI is machine learning based. AI technology is concerned with creating software and hardware capable of performing tasks normally associated with Natural intelligence. That is, an AI uses machine learning systems that can build neural models from examples and minimize the distance between the output and the target.
What does it take for an AI to perform well?
- Powerful computing infrastructure.
- An extraordinary volume of data.
Where does all the data originate?
Where does all the data available today come from? From the surveillance-based business model that large corporations have been using since 2010 (not only Big Tech’s). Surveillance-based model makes it possible to intercept the vast flow of data and metadata that every human activity generates. Large companies have also always had the ability to analyze this data within the computing and processing most technologically advanced infrastructure. Access to data and access to processing infrastructure means unlocking the potential of computing algorithms to achieve ever higher performance.
For example, most smartphones use Google’s Android operating system. One study found that an idle Android phone sent Google 900 data points over the course of 24 hours, including location data. Facebook also tracks users on Android through its apps, logging people’s call and SMS history. [here]
Sub-symbolic AI systems
Cognitive models are an explanation of how some aspect of knowledge is realized by some set of primitive computational processes. So, to make it simple, a symbolic cognitive model is the human’s cognition that take the form of working computer programs. When we talk about AI systems today, we are talking about sub-symbolic AI systems. Traditional AI, the kind we have always known, was symbolic, not sub-symbolic. It was based on Aristotelian logic, which made it always explicable. The advantage of a symbolic system is that it can be based on logical relationships between his parts. Imagine a programmer writing code, and imagine that the program behaves in an unintended way (bug), at which point the programmer checks the code and corrects the bug according to Aristotelian cause-and-effect logic. Quite simple. However, this is not possible with today's machine learning systems, because they are statistical in nature. That is, they build models from examples, they do not do anything particularly intelligent, but use automated statistics to draw correlations. Their "power" today is their reliance on huge computing infrastructures. To sum it up:
- Statistical systems based on powerful computing infrastructures
- Massive data availability
- Algorithms capable of exploiting this data.
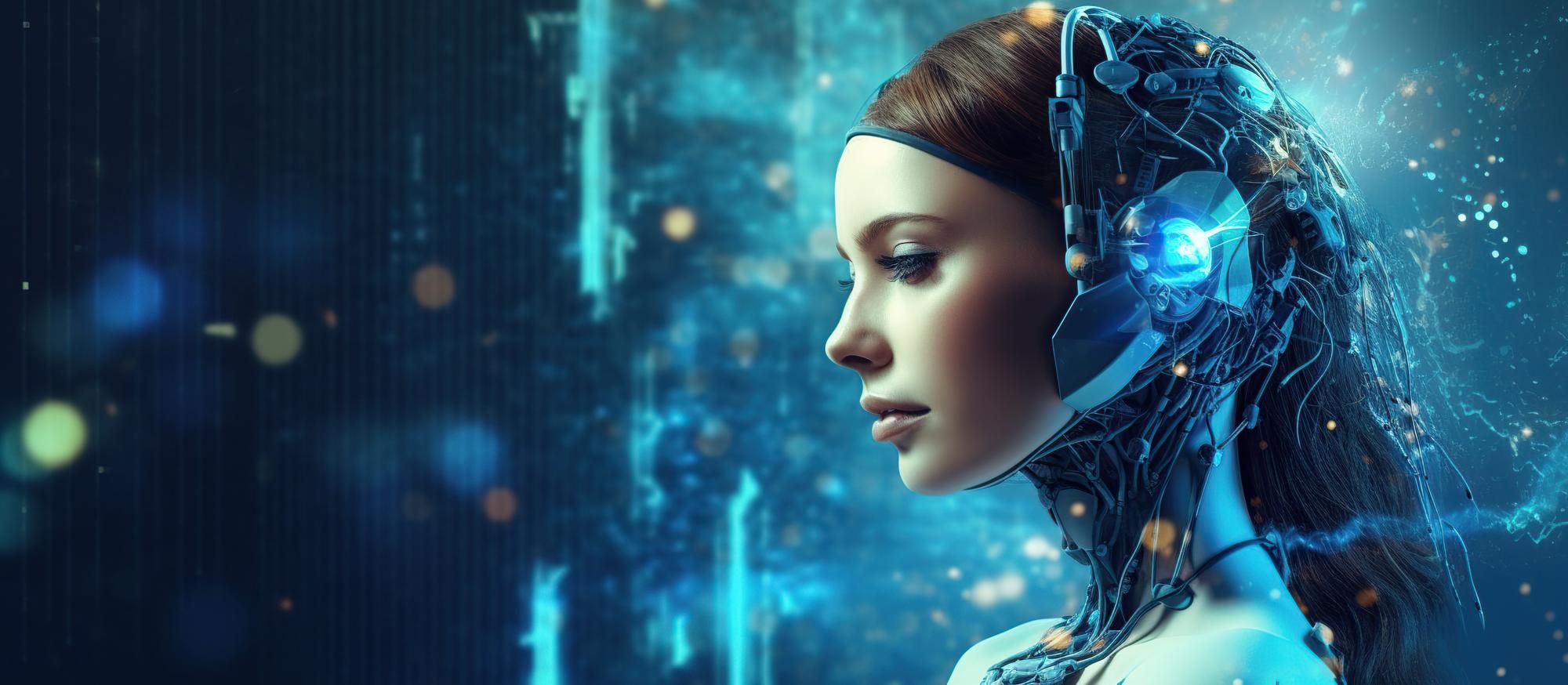
Human Intelligence Value
How do statistical systems operate? If I want to correctly label images of, say, chairs and tables, I have to identify a set of elements that, when added together, allow me to distinguish between them. When we say that an AI learns like a child, we are actually saying something inaccurate, because child does not need millions of labels to distinguish between chairs and tables, it needs very little data and will be able to distinguish them immediately. The child's brain is not statistical. This is true intelligence, human intelligence: the child can do many more things than he is aware of. In fact, we go above and beyond, we humans do more things than we are aware of, or than we can logically explain. We can do things before anyone tells us how to “classify” them. AI seems smarter than a child, or a human brain in general, because it is more powerful, but it is more powerful at the single function, such as generating a drawing. But that's what our calculators did in school when we were trying to pass math tests. Our calculator could do math better than we could, faster, and without error, but we never considered it smarter than us or the people who built it.
Precog beings in Minority Report
A statistical learning system optimizes the output (what the system produces) with respect to a given target. If I give the system a billion labeled photos, the system will draw correlations between the data, but these correlations are not causal, so they're not logical. They're statistical, functional, to minimize the distance to the target. An AI today does not have the ability to make causal connections, and the implication is huge, because establishing causal correlations means using intelligence to understand. Statistical correlations are automation, not understanding. Remember the three precog beings in Minority Report? Their intelligence was statistical, but not causal. They could predict a certain crime based on the possibility that it would actually occur. A possibility that was only considered statistically effective by the system if at least two of the three precogs “saw” it.
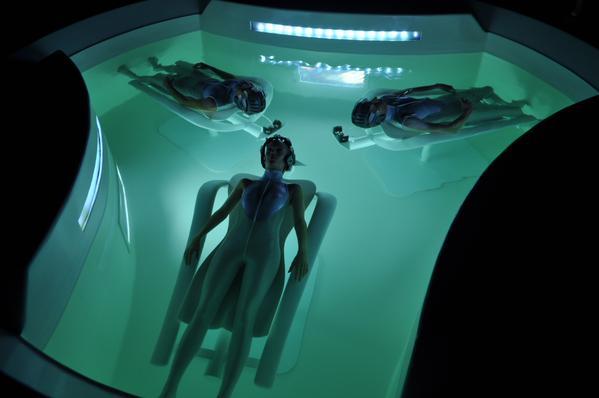
Ethical and unethical AIs?
Are there good and bad uses of AI? Are there ethical and unethical AIs? The big companies had a monopoly on the development of next-generation AI because they were the only ones who could intercept all the most significant data to accumulate enough to repurpose it. And we've allowed them to do that, too. Imagine you go into a car dealership, you pick out a car and you buy it. The dealer says to you, "Take the car, pay for it, but you should know that in order to use it, you have to allow me to stay in constant contact with you through microphones. Then I can know the music you're listening to, who you're listening to it with, when you’re listening to it, how you're feeling, how old you are - either that or no car at all. Wasn’t that what happened with the apps? And what has been the use of all the data that has been accumulated through this mechanism of constant surveillance? How many photos of ourselves have we uploaded to social media platforms? Us at home, us at the beach, us with the girlfriend, us at lunch, us at dinner, us with the newborn child, us with the new car, us at the doctor, us sick, us happy, us sad - us here and us there. We: faces, images, data. When we ask an image-generating AI to portray someone on the beach where does it get its reference examples from? We gave them, over years of more or less conscious surveillance.
Surveillance-based business model
I track your data.
I intercept your conversations.
I index your photos.
After years, how much data have big companies gathered? Enough to profile us with algorithms and to manipulate us to the point where they can determine what we are going to do. And not only that, there is a lot more to it. There is the notion of a hyper-reliable technology with human sensitivity that they want to sell us along with AI. The argument goes something like this: If your AI can reproduce a face so well that it can recognize your own, then maybe it can understand what changes in your face based on your mood. Your AI will have an understanding of when you are sad and when you are happy. Your AI can be better around you than a human friend. Your AI can be the judge of who should be your friend and who should not be. Your AI can make a choice for you.
Dehumanization of humans
In ning these systems as "intelligent" or even "sensitive," one must also consider that the humanization of machines always corresponds to the dehumanization of humans. The infallibility attributed to machines does not exist. The ethics of artificial intelligence, this kind of not better explained ability to discern, mysteriously acquired in deep learning, is not at all able to avoid discrimination in judgment or choice when entrusted to machines (it is well known that AI has been introduced in the courts or in the military). The ethics of artificial intelligence is in fact a commodity that big companies use because they need it. Because research costs money, and infrastructure is expensive, so the message conveyed by those who produce it must instill blind faith in these technologies. But like any other contemporary product (books, movies, novels, songs, video games...), AIs fall under what's called "reputational capital". They fall under marketing, under cultural monopoly. Think of a classic model, one you are probably familiar with: A company that makes a product, markets it, and then responds to its customers about whether the product works well or not. Large high-tech companies, often associated with large universities, have no interest in this model of production and distribution. AIs have been mass-marketed not because they work, but because there is a need for a user base that will use them and begin to believe that they are perfect problem-solving and perfect substitute for human intelligence. They are not, though.
What can be the conclusions?
- We should not think that everything an AI does is correct just because an AI does it. That is not true. It is not a logical system, but statistical.
- It is going to work well in the future because it is a learning system. This is also not true. An AI does not distinguish between right and wrong. It minimizes the distance between target and outcome using statistical correlations.
- There is a linear and increasing growth of AIs, so it is inevitable that they will get better and better. What is the evidence for this? We know that their performance improves based on the amount of data they get, not on understanding the mistakes.
- Technological research and trade are separated. Well, the idea that everything that falls under research is “innovative” in a positive sense is not true. It's a utopia, because much of the research is in the hands of monopolized companies.
- There are no laws that can be put in place for the regulation of AI, its use, and its ethics. And AI does not need external ethical parameters because machine learning will provide them. This is not true, either. If a company produces a system that is not compliant with the laws that exist, then it is violating those laws. Take privacy. When violated, the exiting law defining its absolute value is violated. Trying to solve the problem of violation of laws by proposing other laws that override the previous ones by legalizing the violation is a Catch-22.
Thanks for reading!
Insights
- Andriy Burkov (2019). “The Hundred-Page Machine Learning Book.”
- Nick Bostrom (2016). “Superintelligence: Paths, Dangers, Strategies”.
- Kevin P. Murphy (2022). “Probabilistic Machine Learning: An Introduction (Adaptive Computation and Machine Learning series)”.
- Lise Getoor, Ben Taskar (2019). “Introduction to Statistical Relational Learning (Adaptive Computation and Machine Learning series)”.
- Matthias Schonlau (2023). “Applied Statistical Learning: With Case Studies in Stata (Statistics and Computing)”.
- Noah Giansiracusa (2021). “How Algorithms Create and Prevent Fake News: Exploring the Impacts of Social Media, Deepfakes, GPT-3, and More”.
- John R. Suler (2015). “Psychology of the Digital Age: Humans Become Electric”.
- David Lyon (2006). “Theorizing Surveillance”.